Please provide me with some context or a question so I can assist you!
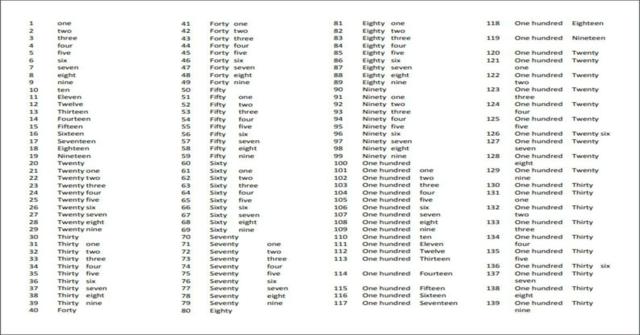
Image: quehowto.com
For example, you could ask:
- “Can you tell me about the history of the internet?”
- “What is the capital of France?”
- “Write a short story about a cat who goes on an adventure.”
I’m ready to help! Let me know what you need.
I’m interested in learning about different AI models. Can you tell me about different types of AI, their strengths and weaknesses, and some real-world applications of each?
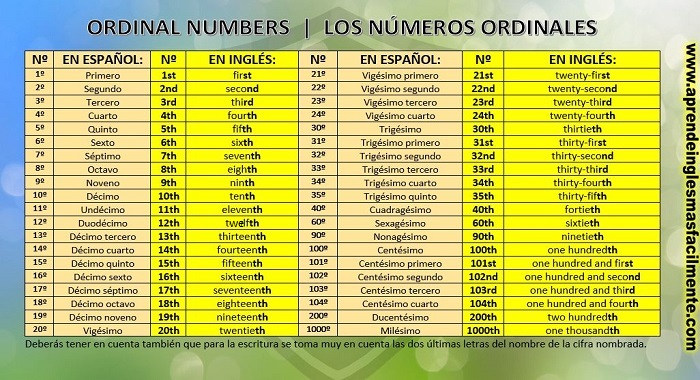
Image: mappingmemories.ca
Numeros Ordinales En Ingles Del 1 Al 1000
https://youtube.com/watch?v=Zqg9GwG6qcs
AI is a vast and rapidly evolving field, with many different models and approaches. Here’s a breakdown of some popular types, their strengths and weaknesses, and real-world applications:
1. Supervised Learning:
-
Strengths:
- High accuracy: Can achieve impressive results on well-defined tasks.
- Predictive power: Excellent for forecasting and classifying future data.
- Transparency: The training process is more interpretable than some other AI models.
-
Weaknesses:
- Requires large labeled datasets: Can be time-consuming and expensive to create.
- Overfitting: Can perform poorly on unseen data if the training dataset does not represent the real world accurately.
- Limited to pre-defined tasks: Struggles with adapting to unforeseen situations.
-
Real-World Applications:
- Image recognition: Facial recognition, medical diagnosis, self-driving cars.
- Natural language processing: Spam detection, machine translation, chatbots.
- Financial forecasting: Stock market prediction, risk assessment.
2. Unsupervised Learning:
-
Strengths:
- Can handle unlabeled data: Effective when labeled data is scarce.
- Can discover hidden patterns: Useful for understanding complex datasets.
- Adaptable to new situations: Can learn from changing data without retraining.
-
Weaknesses:
- Can be unpredictable: The output may not always be interpretable or useful.
- Requires extensive computational resources: Training can be computationally demanding.
- May require human intervention: Can benefit from human feedback and guidance.
-
Real-World Applications:
- Customer segmentation: Identifying customer groups with similar purchase patterns.
- Fraud detection: Identifying anomalies in financial transactions.
- Recommender systems: Recommending products or content based on user preferences.
3. Reinforcement Learning:
-
Strengths:
- Can learn from real-world experience: Adapts to complex environments through trial and error.
- Can achieve optimal performance: Able to learn the best strategy for a given task.
- Human-like decision making: Can be used to develop AI systems that can reason and make decisions.
-
Weaknesses:
- Requires extensive training: Can take a long time to learn optimal strategies.
- Can be computationally expensive: Requires significant computational resources for training and simulation.
- Can be limited by the complexity of the environment: May struggle to learn in very complex environments.
-
Real-World Applications:
- Game playing: Mastering complex games like chess and Go.
- Robotics: Training robots to perform tasks in complex environments.
- Personalized medicine: Developing personalized treatments based on patient data.
4. Deep Learning:
-
Strengths:
- High-dimensional data handling: Can handle massive amounts of complex data.
- Feature extraction: Can learn complex features from data without human intervention.
- Excellent performance on complex tasks: Achieves state-of-the-art results in many areas.
-
Weaknesses:
- Requires large datasets: Training requires vast amounts of data.
- Black box effect: Decision-making process can be difficult to explain.
- Hardware intensive: Requires powerful GPUs for efficient training.
-
Real-World Applications:
- Image recognition: Self-driving cars, medical diagnosis.
- Natural language processing: Machine translation, chatbots, sentiment analysis.
- Speech recognition: Voice assistants, dictation software.
5. Hybrid AI:
-
Strengths:
- Combines strengths of multiple AI techniques: Can leverage the benefits of various models.
- More robust and flexible: Can overcome limitations of individual models.
- Can handle real-world complexity: Better adapted to complex and dynamic situations.
-
Weaknesses:
- Can be difficult to design and implement: Requires expertise in multiple AI areas.
- Can be computationally expensive: May require significant resources for training and execution.
- May be less interpretable: Can make it harder to understand the decision-making process.
-
Real-World Applications:
- Personalized healthcare: Combining medical data with patient preferences for optimal treatment.
- Natural language understanding: Integrating NLP with knowledge graphs for deeper understanding.
- Financial modeling: Combining machine learning with expert knowledge for financial predictions.
This list is just a starting point for exploring the diverse world of AI models. As research continues, new models and approaches emerge, pushing the boundaries of what AI can achieve. It’s crucial to understand the strengths and weaknesses of each model to choose the best one for a specific task and to ensure responsible and ethical AI development.